The Florida Museum of Natural History recently welcomed Arthur Porto as its first curator of artificial intelligence for natural history and biodiversity. Prior to joining the museum, Porto was an assistant professor of biology at Louisiana State University where he used machine learning and image-based technology to study the tempo of evolution. In his new role, he plans to expand on these techniques and use them to identify hidden patterns in brimming digital repositories of natural history data.
Porto has had a fascination for biodiversity and natural history museums since childhood, when he dreamed of becoming a paleontologist. But a competing interest in mathematics and machine learning pulled him in a different direction, one that made a museum-based career seem unlikely. Rather than specializing in a specific group of organisms — often a pre-requisite for museum curators — Porto developed a chimeric expertise focused on methodology.
“I’ve always wanted to be a curator, but with the way museums are traditionally organized, I thought I’d have to stick to a biology or a computer science department,” Porto said. “But when this positioned opened, it was for someone to do the same things I was already working on.”
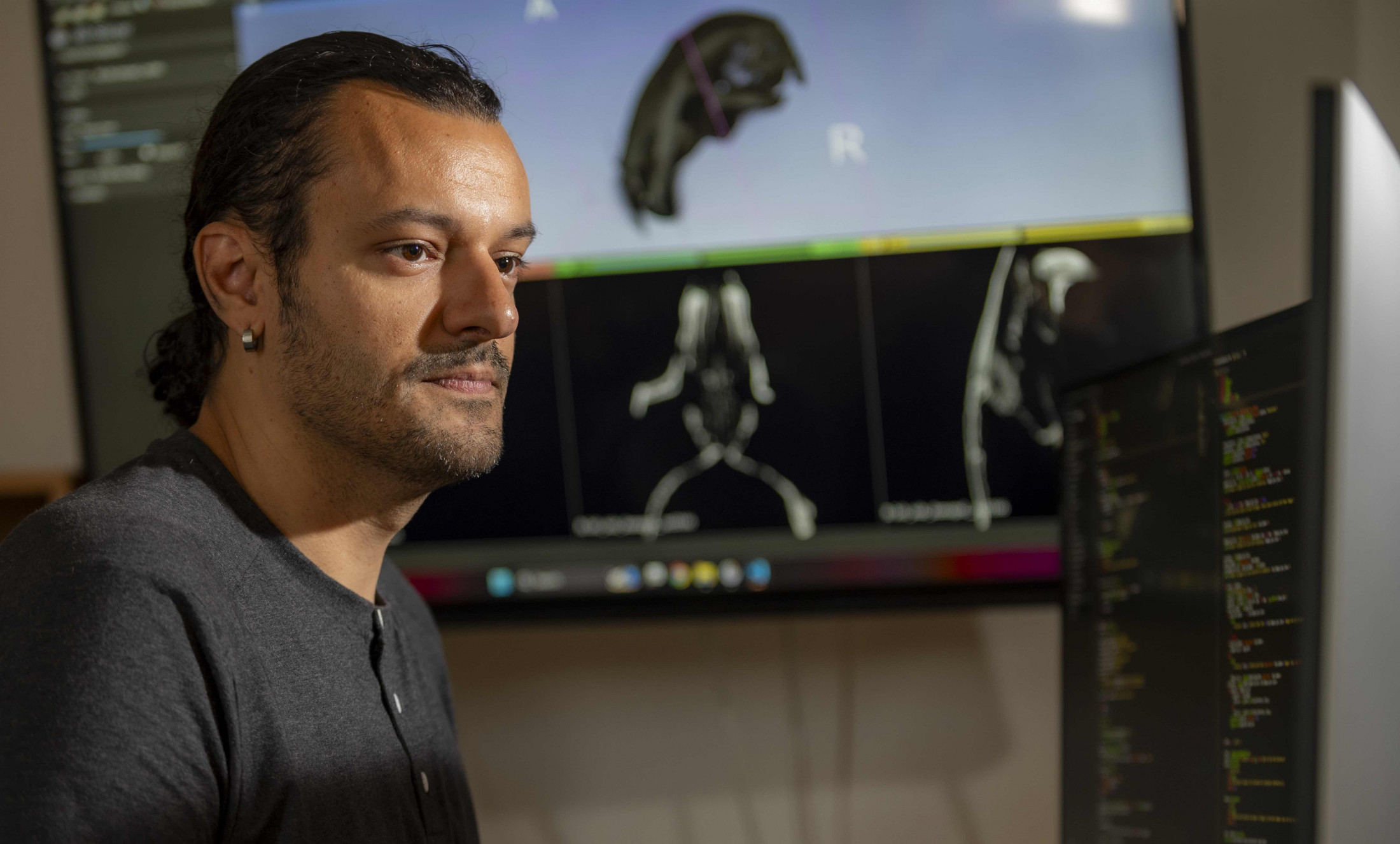
Florida Museum photo by Kristen Grace
The job is one of several recently created at the University of Florida with a focus on artificial intelligence. In 2020, the university launched an initiative to cement its reputation as a world leader in AI research and integrate machine learning concepts and courses into its curriculum.
Porto has, for his part, spent his career developing the technology that helped make jobs like these possible. While working toward a bachelor’s degree in biology at the University of São Paulo, he used what was considered advanced technology at the time to create digital reconstructions of mammal skulls.
“I used a mechanical arm, which held a pen and kept track of its position relative to the arm’s base. You had to take the pen, place it on the specimen in different positions and press a button for a computer to record the three-dimensional coordinates,” he said.
By comparing the same skull features across multiple species, Porto could make inferences about how animals evolved. But the process of placing landmarks to create a 3D representation was laborious and expensive. Porto had to take the robotic arm with him to each museum that housed a skull he wanted to measure, severely hampering the amount of work he could accomplish for his degree.
By the time he finished his Ph.D. a few years later, museums had begun using CT scanners to create 3D specimen reconstructions. This was an immeasurable improvement to the disembodied arm approach, but anyone hoping to use these digital replicas for comparative research still needed to place landmarks on all the 3D models they wanted to study.
So Porto created a program called ALPACA that automated the process. “Now, instead of having to manually measure hundreds of specimens, you can measure, let’s say, four and use them as templates. The landmarks on those templates are automatically fitted to any other specimen you want to measure.”
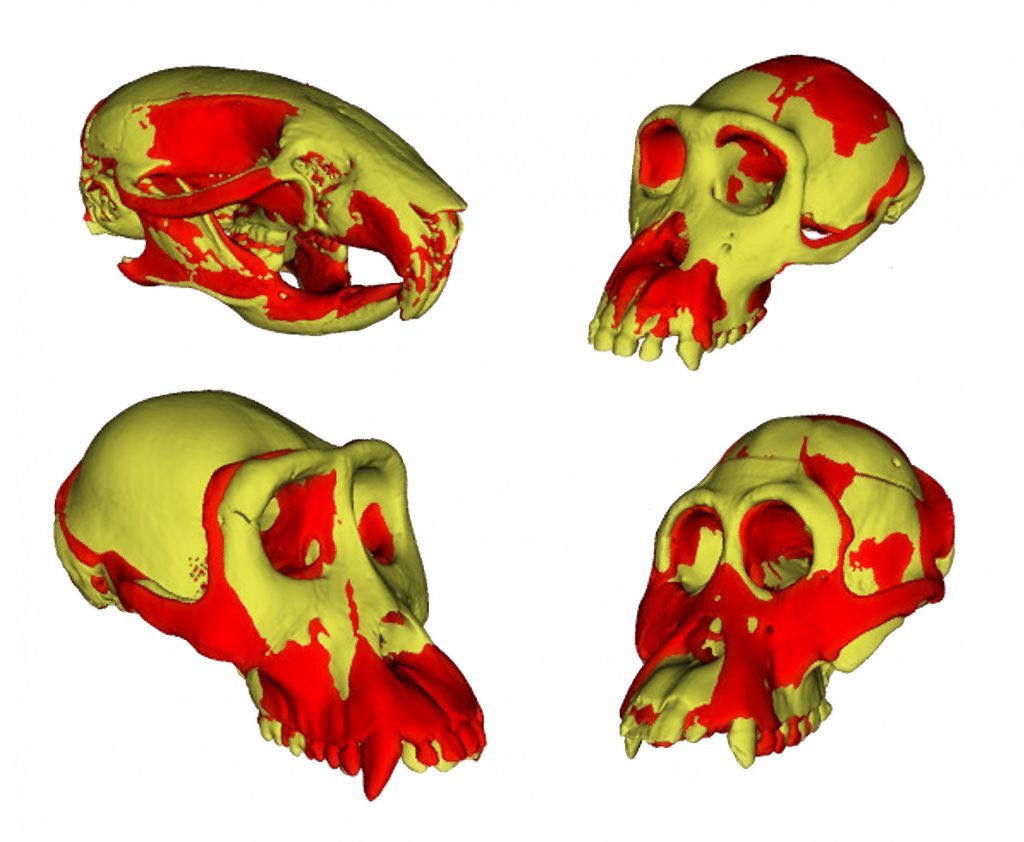
Image courtesy of Arthur Porto
This wasn’t the first program he created to scale up the acquisition of data. For his second postdoctoral fellowship, Porto joined a lab in Norway that was trying to crack an evolutionary mystery called the “paradox of stasis.” Whenever a population of organisms is faced with a change in their environment, they have two options: adapt or die out. Natural history is replete with the latter — species that couldn’t keep up and went extinct as a result — but there is also no shortage of winners that were able to successfully change in response to external forces. The records of those that survived prove organisms can evolve quickly, yet on the vast scale of geologic time, species seem to change at a rate much slower than the one they’re capable of.
To really study this problem, you need organisms with an exceptional fossil record, and there are only a few groups that fit the bill. Bryozoans, a type of marine invertebrate, are one such exception. “You can literally go to a fossil site and dig up millions of them in a short period of time,” Porto said.
Previous fossil studies seemed to confirm bryozoans adhered to a mode of punctuated equilibrium, evolving in short bursts, then settling down for eons of stasis. Porto wanted to take another look, but processing the fossils using traditional methods would require countless hours of mind-numbingly meticulous work. Bryozoans are colonial animals that consist of individual units called zooids, which have a variety of unique functions and features. An electron micrograph of a single bryozoan can consequently contain hundreds of structures that only a trained expert can identify.
“I actually put pen to paper and calculated the amount of time it would take me to collect data for the project,” Porto said. According to his estimates, he’d need to work roughly 200, eight-hour days to complete the task.
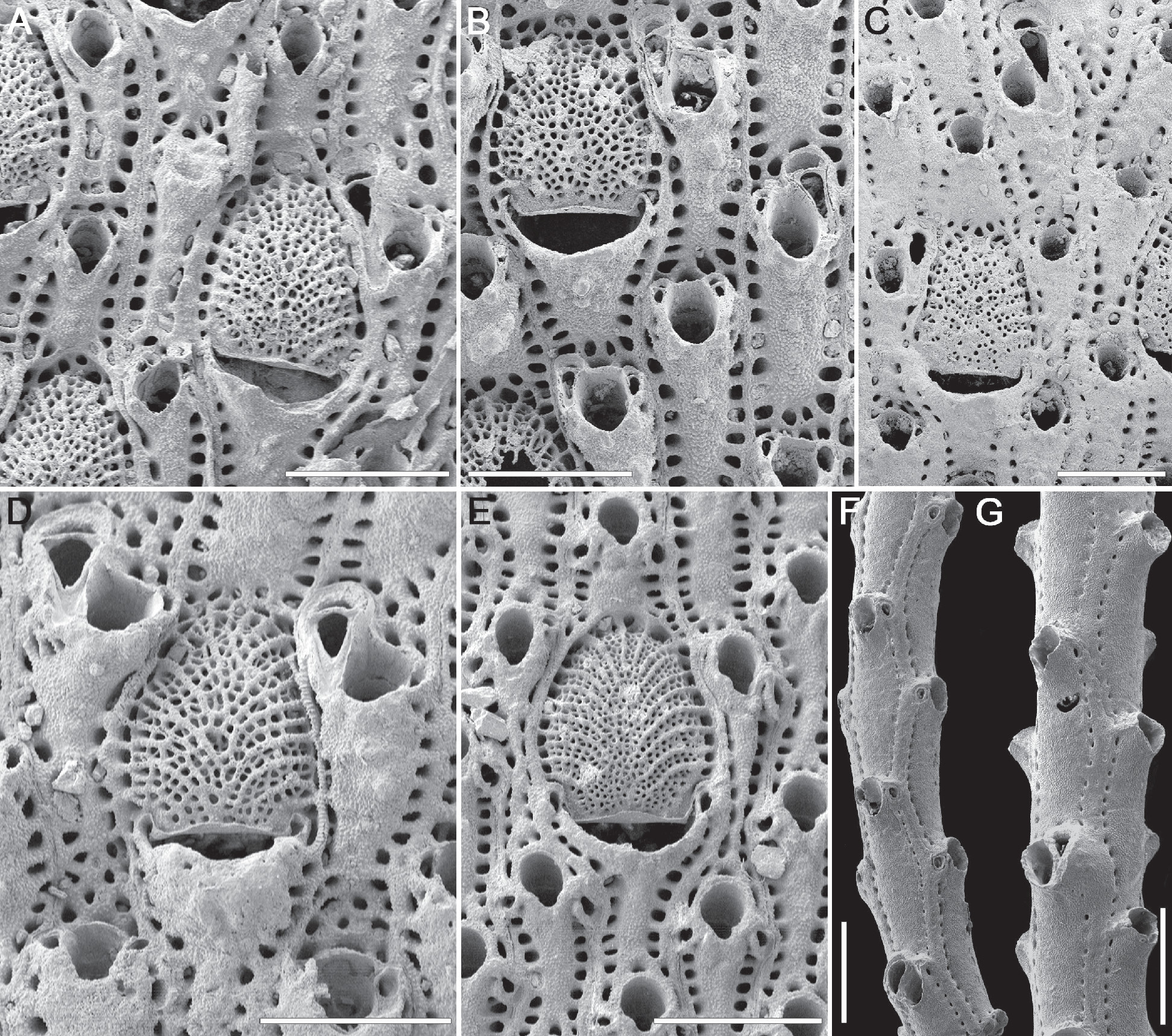
Image by Vole et al., 2020 in The American Naturalist
Repetitious pattern matching, while arduous for humans, is exactly the thing machine learning excels at. Porto developed a program called Deep Bryo that could do the work for him in a fraction of the time — instead of 200 days, the program collected the same amount of data in one hour.
This type of machine learning, called computer vision, has been widely adopted by the medical community, where it’s used to help make diagnoses. In fact, Porto had the idea for Alpaca while working at the Seattle Children’s Hospital, where he’d been hired to study the cranial traits of mice.
But the potential of computer vision is only just now being recognized in evolutionary biology, Porto said. Similar to the ongoing revolution in genomics — the study of an organism’s full complement of DNA — Porto thinks biology is on the cusp of a similar revolution in phenomics, in which images are used to study the sum total of an organism’s physical traits. “Now we can collect image data much faster than we can process it,” he said.
Porto is currently recruiting graduate students with a background in biology or computer science. “The big focus of my lab is method development, creating new approaches to study organisms, including methods where you’re not just asking for specific things that you want from the data, but also trying to interpret how the models are learning to begin with.”
Source: Arthur Porto, arthur.porto@ufl.edu
Writer: Jerald Pinson, jpinson@flmnh.ufl.edu, 352-294-0452